Decoding Emotions: A Deep Dive into the Power of Sentiment Analysis
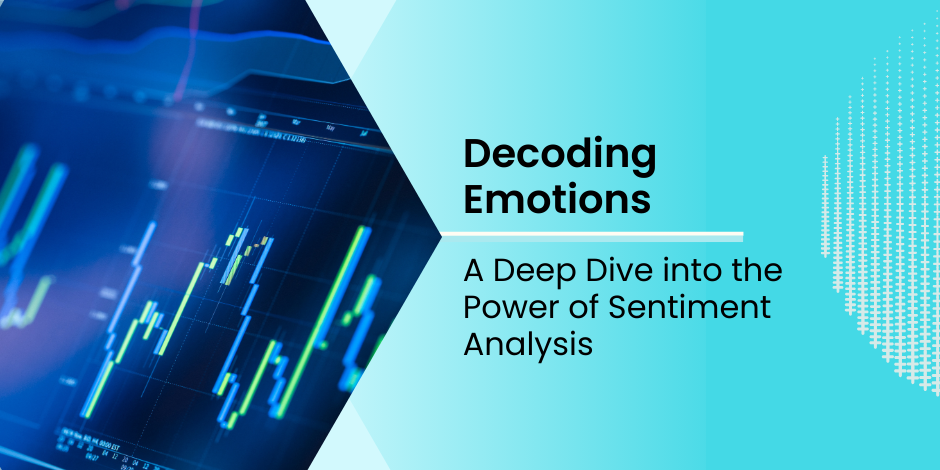
Stay Informed With Our Weekly Newsletter
Receive crucial updates on the ever-evolving landscape of technology and innovation.
Sentiment analysis, or opinion mining, is a wide-reaching technique for understanding and interpreting human emotions expressed in textual data.
It involves using machine learning (ML) and natural language processing (NLP) to analyze and classify the sentiment or tone of a piece of text, whether it is positive, negative, or neutral.
Let’s explore the power of sentiment analysis and its importance in today’s digital world.
Understanding sentiment analysis
The science behind the power of sentiment analysis
At its core, sentiment analysis is a process of computationally identifying and categorizing subjective information conveyed in text data.
It requires an understanding of linguistics, semantics, and ML techniques.
Sentiment analysis algorithms are trained using large datasets that contain labeled examples of positive and negative sentiment.
These training datasets allow the algorithms to learn patterns and associations between words and sentiment.
Sentiment analysis models can then accurately predict the sentiment of new, unseen pieces of text using ML techniques.
The process of sentiment analysis involves several steps:
- The text data is preprocessed by removing unnecessary punctuation, stop words, and special characters.
- The preprocessed text is tokenized into individual words or phrases.
- The sentiment analysis model assigns sentiment scores to each token based on context and meaning.
- These token-level sentiment scores are aggregated to determine the overall sentiment of the text.
The importance of sentiment analysis in today’s world
Sentiment analysis is becoming increasingly important due to the explosive growth of social media and online content.
People freely express their opinions and emotions on various online platforms, leaving behind a vast amount of valuable data that can be analyzed and leveraged.
Organizations can use sentiment analysis to gain insights into customer sentiment, public opinion, and emerging trends, giving them an edge and allowing them to make data-driven decisions.
The process of sentiment analysis
How does sentiment analysis work?
The power of sentiment analysis involves a two-step process: training and applying the model to new data.
During the training phase, sentiment analysis algorithms learn from labeled datasets containing positive and negative sentiment examples.
These labeled examples allow the model to understand patterns and associations between words and sentiments.
Once trained, the model can be applied to new, unseen text data.
The text data is preprocessed by removing noise and irrelevant information.
Then, the model assigns sentiment scores to each token based on context and meaning.
These sentiment scores are often represented on a scale ranging from -1 for extremely negative sentiment to +1 for extremely positive sentiment.
The role of artificial intelligence (AI) in sentiment analysis is crucial.
ML algorithms, particularly deep learning models such as recurrent neural networks (RNN) and transformer models, have shown remarkable performance in sentiment analysis tasks.
These AI models can capture complex patterns and long-term dependencies and understand the context of the text, leading to highly accurate sentiment predictions.
The role of AI in sentiment analysis
AI has revolutionized the power of sentiment analysis by enabling more accurate and efficient sentiment classification.
AI models, particularly deep learning models, can learn from large datasets and capture intricate patterns and nuances in language.
Deep learning models, such as RNN and transformer models, have shown remarkable performance in sentiment analysis tasks.
These models can understand the language’s context, capture temporal dependencies, and effectively handle variations in expression and tone.
They can process large amounts of text data and learn the underlying sentiment patterns, resulting in highly accurate sentiment predictions.
The role of AI in sentiment analysis extends beyond classification.
By analyzing sentiment data over time, AI models can identify emerging trends, detect anomalies, and provide valuable insights into the dynamics of public sentiment.
Challenges in sentiment analysis
The complexity of human emotions
Human emotions are complex and multifaceted and often difficult to quantify accurately.
Sentiment analysis algorithms struggle to capture the full spectrum of emotions expressed in text data, particularly the subtle variations, and nuances humans can easily recognize.
Sarcasm, irony, and other figurative language pose significant challenges in sentiment analysis, as their meaning may be context-dependent or contrary to the literal interpretation.
Another challenge lies in the cultural and contextual variations of sentiment expression.
Different cultures and groups may have different ways of expressing and interpreting emotions, making it difficult to build universal sentiment analysis models.
Dealing with sarcasm and irony in sentiment analysis
Sarcasm and irony are prevalent forms of communication in online platforms, making them critical challenges for sentiment analysis.
These forms of figurative language involve expressing the opposite of what is meant or using words with a different intended meaning.
As a result, sentiment analysis algorithms may misinterpret sarcasm or irony as genuine, leading to inaccurate sentiment classifications.
Applications of sentiment analysis
Sentiment analysis in business
In business, understanding customer sentiment is crucial for success.
The power of sentiment analysis allows companies to monitor customer opinions, reviews, and feedback, enabling them to identify areas for improvement, track brand reputation, and develop effective marketing strategies.
By analyzing social media platforms and customer reviews, businesses can gain valuable insights into customer satisfaction, product preferences, and emerging trends, helping them stay competitive.
Sentiment analysis can also be used to analyze market trends and make predictions.
Businesses can detect emerging trends by analyzing social media conversations and news articles, identifying potential market opportunities, and adapting their strategies accordingly.
Sentiment analysis in social media monitoring
Social media platforms are goldmines of information regarding public sentiment.
Sentiment analysis allows companies and governments to monitor social media channels to understand how the public perceives their brand, products, or policies.
Sentiment analysis can help detect and manage potential crises, track sentiment toward specific topics, and understand public sentiment during major events or elections by analyzing social media conversations.
Sentiment analysis on social media is not limited to brands and politics only.
It also has applications in detecting and preventing online harassment, cyberbullying, and hate speech.
The power of sentiment analysis models helps to identify toxic or negative comments, enabling social media platforms to take appropriate actions to ensure a safe and positive online environment.
The future of sentiment analysis
Emerging trends in sentiment analysis
One emerging trend in sentiment analysis is integrating multimodal data, where sentiment analysis algorithms analyze text and non-textual data such as images, videos, and audio.
By incorporating visual and auditory information, sentiment analysis models can capture a more comprehensive picture of sentiment and emotion, enabling more accurate and nuanced sentiment analysis.
Another trend in sentiment analysis is using transfer learning and pre-trained language models.
Transfer learning allows sentiment analysis models to leverage knowledge gained from one task to improve performance on another related task.
The potential impact of advancements in AI on sentiment analysis
Advancements in AI, particularly in areas such as deep learning and natural language generation, are expected to profoundly impact the power of sentiment analysis.
These advancements will lead to more accurate sentiment analysis models, better handling of complex emotions and figurative language, and improved adaptability across different cultures and domains.
Additionally, advancements in AI will enable sentiment analysis models to process and analyze larger volumes of data in real time, providing organizations with real-time insights into customer sentiment, emerging trends, and potential issues.
Conclusion
Sentiment analysis holds immense value in understanding and decoding human emotion in the vast sea of daily textual data.
The power of sentiment analysis enables businesses, governments, and organizations to gain crucial insights into customer sentiment, public opinion, and emerging trends by employing sophisticated techniques and leveraging AI.
As technology advances, the power of sentiment analysis will continue to evolve, providing even more accurate, real-time, and nuanced analysis of the complex tapestry of human emotions.
Considering a data science career?
Choose the Institute of Data’s Data Science & AI Program for an in-depth, balanced curriculum designed to prepare you with the skills you’ll need for success in this competitive field of tech.
Learn more about our 3-month full-time or 6-month part-time programs by reading the Data Science & AI Course Outline.
Ready to learn more about our programs? Contact our local team for a free career consultation.