How Long Does it Take to Become a Data Scientist?
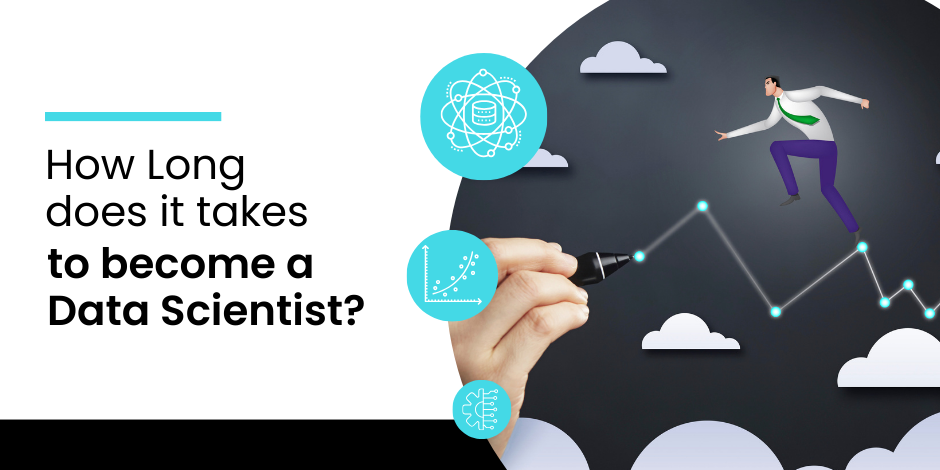
Stay Informed With Our Weekly Newsletter
Receive crucial updates on the ever-evolving landscape of technology and innovation.
Becoming a data scientist is an enticing career path, given the exponential growth of data and its increasing influence on decision-making across industries.
But, no matter how long it takes to become a data scientist, one thing is certain: it is key to remember that it’s not a sprint but a marathon.
Become A Data Scientist: Understanding the role
Data scientists extract actionable insights from complex data sets.
Their responsibilities extend beyond data analysis; they also possess a thorough understanding of the business domain and the ability to communicate their findings effectively.
Become A Data Scientist: Core skills
The core skills required for a data scientist include proficiency in programming, data wrangling, statistical analysis, and machine learning (ML).
Data scientists are at the forefront of leveraging data to solve complex problems, drive innovation, and enable evidence-based decision-making.
One key aspect of a data scientist’s role is the ability to work with large and diverse datasets, often collected from various sources.
Data scientists must be adept at cleaning and preprocessing data to ensure its quality and reliability for analysis.
Data scientists must be adept at collaborating with cross-functional teams to understand the specific requirements of a project and align their data analysis efforts with the overall business goals.
Become A Data Scientist: Educational journey
Embarking on the educational path to become a data scientist involves a combination of formal education, self-study, and practical experience.
While there is no prescribed route, earning a degree in data science or a related field can provide a solid foundation.
Universities now offer dedicated data science programs that equip students with comprehensive skills, including statistics, programming, and data manipulation.
Studying mathematics, computer science, and economics can also provide a solid theoretical foundation for data science.
However, formal education is only the beginning.
To become a well-rounded data scientist, continuous learning is vital.
An accredited certification like the Institute of Data’s Data Science & AI program is a popular alternative to formal education.
Gaining practical experience through certifications, internships, research projects, or industry collaborations can significantly enhance a data scientist’s skill set.
Working on real-world data sets and solving complex problems can provide invaluable insights that cannot be gained in a classroom setting alone.
Networking with professionals in the field and attending conferences, workshops, and meetups can also help aspiring data scientists stay connected with industry trends and build a strong professional network.
Collaborating with experts from diverse backgrounds can offer new perspectives and foster innovation in data science projects.
Become A Data Scientist: Qualifications and Certifications
To become a data scientist, you might consider enhancing your credentials by obtaining relevant certifications.
Certifications validate the knowledge and skills required for specific data science techniques and tools.
Continuous professional development through workshops and conferences also plays a crucial role in honing data science skills.
Data scientists can distinguish themselves by participating in hackathons and data science competitions.
These events provide a platform to showcase practical skills and offer opportunities to network with industry professionals and potential employers.
Winning or participating in such competitions can significantly boost a data scientist’s profile and credibility within the industry.
Attending meetups, joining online forums, and engaging in discussions on platforms like GitHub can help data scientists stay updated on the latest trends, tools, and techniques in the field.
Building a network of mentors and peers can provide valuable insights and support in navigating the complexities of data science projects and career progression.
Become A Data Scientist: Practical experience
While education and certifications are important, practical experience is equally invaluable.
Internships and entry-level jobs allow aspiring data scientists to apply their skills in real-world scenarios and gain industry experience.
Many organisations offer data science internships, and these valuable learning experiences can serve as a stepping stone to employment.
Hands-on experience with datasets, real-world problems, and industry tools helps bridge the gap between theory and practice.
It also enables aspiring data scientists to build projects that showcase their abilities to potential employers.
Practical experience in data science allows individuals to develop important soft skills such as communication, teamwork, and problem-solving.
These skills are essential for collaborating with colleagues, presenting findings to stakeholders, and effectively tackling complex data challenges professionally.
Working on real projects exposes data science enthusiasts to data collection, cleaning, and analysis nuances.
Understanding the intricacies of data preparation and manipulation is fundamental in ensuring the accuracy and reliability of insights derived from datasets.
Become a Data Scientist: The timeline
The time required to become a skilled data scientist varies depending on several factors, including prior knowledge, educational background, and dedication to the journey.
While some individuals may be able to transition into the field relatively quickly, others require more time and effort.
To become a data scientist, it’s important to note that everyone’s journey is unique, and the time frame may differ based on individual circumstances.
To provide some guidance, let’s examine a realistic timeline:
- Year 1: Get started with foundational courses, such as mathematics, statistics, and programming.
- Year 2: Pursue a degree, program, or certification in data science or a related field while expanding your knowledge of ML and data visualization.
- Year 3: Gain practical experience through internships or entry-level positions. Start building a portfolio of projects.
- Year 4: Continuously update skills and delve deeper into specialized areas of interest, such as natural language processing or computer vision.
- Year 5: Advance in the field by taking on more complex projects and contributing to the data science community through publications or open-source contributions.
Remember, this timeline is not set in stone and can vary based on individual circumstances.
Conclusion
To become a data scientist, you will need a solid education, practical experience, and continuous learning.
While the journey may be demanding, the rewards are substantial.
By acquiring the necessary skills, staying updated with industry trends, and gaining practical experience, individuals can carve a successful career in this exciting field.
So, if you’re fascinated by data and possess a curious mind, embark on your journey to become a data scientist today.
Are you ready to dive into data science?
The Institute of Data’s Data Science & AI program offers an in-depth, balanced curriculum and flexible learning options taught by industry professionals.
Join us to enhance your job prospects in this fascinating, dynamic field of tech.
Ready to learn more about our programs? Contact our local team for a free career consultation.