How to Build a Data Science Portfolio
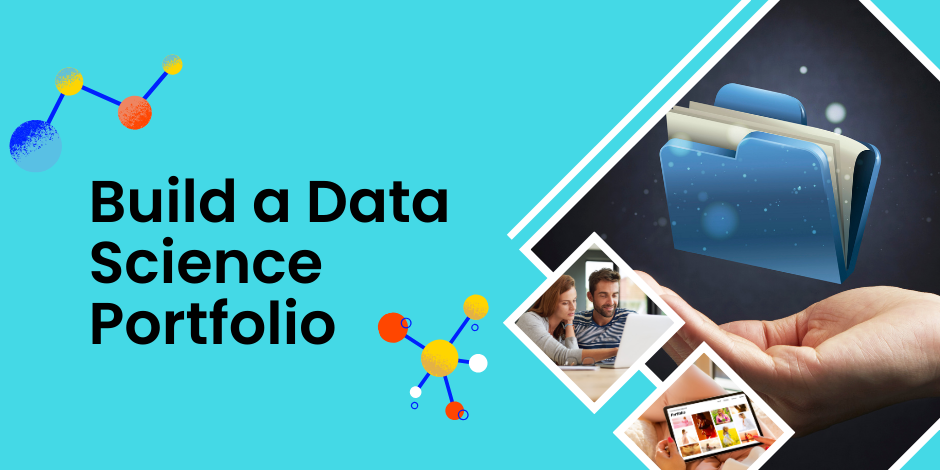
Stay Informed With Our Weekly Newsletter
Receive crucial updates on the ever-evolving landscape of technology and innovation.
Data science is a rapidly growing field with a high demand for skilled professionals.
As a data scientist, building a data science portfolio is one of the most effective ways to showcase your expertise and stand out from the competition.
Understanding the importance of a solid portfolio
A robust portfolio serves as a visual representation of your skills, accomplishments, and problem-solving capabilities.
It allows potential employers or clients to assess your proficiency in handling real-world data science projects.
A well-crafted portfolio can demonstrate your ability to apply data analysis techniques, communicate insights, and create meaningful visualisations.
When it comes to data science, theory alone is not enough. Employers and clients want to see practical examples of your work.
They want to know that you can take raw data and turn it into actionable insights.
That’s where a strong portfolio comes in.
It provides tangible evidence of your abilities and allows you to showcase your expertise beyond your resume or qualifications.
The role of a portfolio in a data science career
A portfolio is a collection of projects and a testament to your expertise and dedication to the field.
It provides concrete evidence of your capabilities, allowing you to showcase your skills beyond theoretical knowledge.
A well-appointed portfolio proves your ability to solve complex problems using statistical analysis, machine learning algorithms, and data visualisation techniques.
Furthermore, a data science portfolio can help you stand out in a competitive job market.
With the increasing demand for data scientists, employers seek candidates who can demonstrate their skills and differentiate themselves from others.
A well-curated portfolio can make you a more attractive candidate and increase your chances of landing your dream job.
Key elements of an effective portfolio
An effective portfolio should include the following key elements:
- Relevant and diverse projects that demonstrate your expertise in various data science domains.
- Clean and well-documented code that showcases your programming skills.
- Clear and concise project descriptions that highlight the problem, methodology, and results.
- Engaging visualisations that effectively communicate insights.
- Links to relevant datasets, GitHub repositories, or deployed applications.
When selecting projects for your portfolio, choosing ones that showcase your skills in different areas of data science is essential.
This demonstrates your versatility and ability to tackle a wide range of challenges.
Additionally, provide clean, well-documented code so others can easily understand and replicate your work.
Project descriptions should be clear and concise, providing an overview of the problem you were trying to solve, the methodology you used, and the results you achieved.
Finally, engaging visualisations are crucial for effectively communicating insights.
Data can be complex, and visualisations help simplify and convey information in a more digestible format.
Whether it’s interactive dashboards, charts, or graphs, make sure your visualisations are visually appealing and easy to interpret.
Remember to include links to relevant datasets, GitHub repositories, or deployed applications.
This allows others to explore your work in more detail and see the practical applications of your projects.
Starting your data science portfolio
When starting your portfolio, choosing projects that align with your interests and showcase your skills effectively is important.
Here are some tips to help you get started:
Choosing the right projects for your portfolio
Consider selecting projects that align with your career goals and demonstrate your expertise in specific areas of data science.
Choose projects that involve real-world datasets and require you to apply various data science techniques.
For example, you could develop a predictive model or analyse a large dataset to uncover actionable insights.
Tools and platforms for building your portfolio
There are several tools and platforms available that can help you build and showcase your portfolio.
Popular options include GitHub, Kaggle, and personal websites.
These platforms allow you to upload your projects, share your code and findings, and interact with the data science community.
Structuring your data science portfolio
Effective structuring of your data science portfolio is crucial for engaging potential employers or clients. Here are some strategies to consider:
Organising your projects effectively
Organise your projects logically and coherently, grouping similar tasks.
Consider creating separate sections for different data science domains, such as machine learning, data visualisation, and natural language processing.
This will allow visitors to navigate your portfolio quickly and assess your expertise in specific areas.
Highlighting your skills and achievements
In each project description, highlight the skills and techniques you utilised and any notable achievements.
Explain the problem you tackled, the methodologies you used, and the insights you discovered.
Include visualisations, code snippets, and links to the project source code or live demos.
Enhancing your portfolio with personal branding
Personal branding can significantly impact a competitive field like data science.
Here are some tips to enhance your portfolio with personal branding:
Creating a unique identity for your portfolio
Develop a unique visual identity for your portfolio that reflects your personality and professionalism.
Use consistent design elements, colours, and typography across your projects.
This will help your portfolio stand out and create a memorable impression.
Communicating your brand through your portfolio
Your portfolio should reflect your brand and showcase your unique strengths.
Consider including a brief bio or mission statement highlighting your passion for data science and career objectives.
Use your projects and project descriptions to tell a cohesive story about your skills and accomplishments.
Maintaining and updating your data science portfolio
A successful data science portfolio is dynamic; it should evolve and adapt over time.
Here’s how you can maintain and update your portfolio:
Regularly adding new projects to your portfolio
As you complete new data science projects, add them to your portfolio regularly.
This demonstrates that you are actively learning and applying new skills. Be selective and showcase only your best and most relevant work.
Keeping your portfolio relevant in the changing data science landscape
Data science is a field that constantly evolves and changes.
To give yourself the best chance of success, remember to:
- Stay up-to-date with the latest tools and industry trends to ensure your portfolio remains relevant.
- Revisit your older projects and update them with new insights or methodologies.
- Remove any outdated or irrelevant projects to keep your portfolio focused and impactful.
Conclusion
Building a data science portfolio requires time, effort, and dedication, but it’s a worthwhile investment in your career.
By effectively demonstrating your skills and accomplishments, you can increase your chances of landing exciting data science opportunities and advancing your professional journey.
Ready to launch your data science career?
The Institute of Data’s Data Science & AI program offers a balanced, in-depth curriculum tailored to today’s industry demands.
Choose us as your learning partner to gain the expertise you’ll need with hands-on projects, strong industry connections and real-world scenarios.
Want to learn more about our programs? Contact our local team for a free career consultation.