Math in Data Science: How much is there?
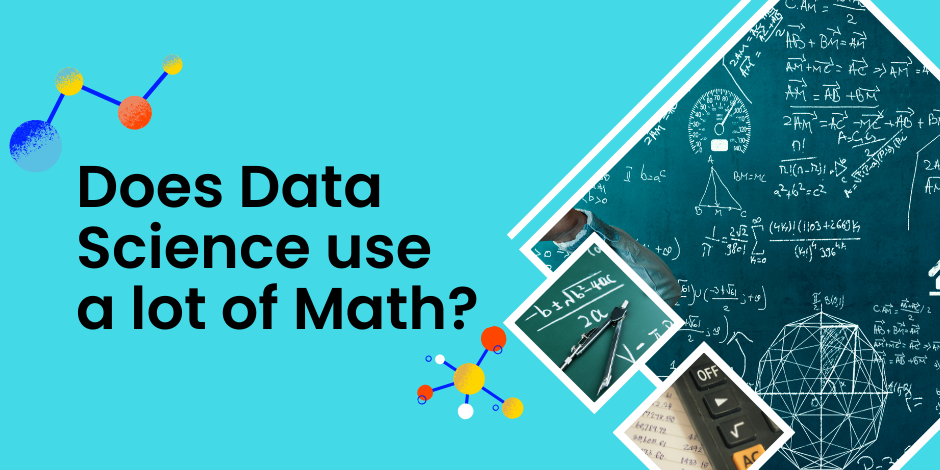
Stay Informed With Our Weekly Newsletter
Receive crucial updates on the ever-evolving landscape of technology and innovation.
When delving into the world of data science, one must recognise the crucial role mathematics plays.
The field is deeply intertwined with various mathematical concepts, making it an essential tool for data scientists.
But how much math is in data science?
This question often arises among those considering a career in this field or those simply curious about the subject.
Let’s delve into the depths of math in data science.
The role of math in data science
Mathematics is the backbone of data science.
It provides the theoretical framework that allows data scientists to extract meaningful insights from complex data sets.
With a solid understanding of the mathematical principles, making accurate predictions or creating effective algorithms would be easier.
Moreover, mathematics is not just a tool used in data science – it is part of its essence.
Data science is built upon mathematical theories and principles, making it a fundamental discipline component.
Therefore, understanding the level of mathematics involved in data science is crucial for anyone considering this career path.
How much math is in data science?
The amount of math in data science can vary depending on the specific role and the nature of the work.
However, at its core, data science heavily relies on several critical areas of mathematics: statistics, calculus, linear algebra, and probability theory.
Statistics is the most apparent mathematical discipline involved in data science. It provides:
- The tools for understanding data.
- Making predictions.
- Making decisions based on data.
On the other hand, calculus and linear algebra are essential for understanding machine learning algorithms, while probability theory is crucial for making sense of uncertain or random phenomena.
Delving deeper into the math in data science
Now that we have a general understanding of the mathematical disciplines involved in data science, let’s delve deeper into each one and explore how they are used in the field.
Math in data science: statistics
Statistics is a branch of mathematics that deals with data collection, analysis, interpretation, presentation, and organisation.
In data science, statistics is used to understand and interpret data, make predictions, and make informed decisions.
For example, descriptive statistics summarise and describe data, while inferential statistics are used to draw conclusions and make predictions based on data.
Furthermore, statistical models represent complex phenomena and provide a basis for machine learning algorithms.
Math in data science: calculus
Calculus, specifically differential calculus, is another key area of mathematics used in data science.
It is used to understand how changes in one variable affect another, which is crucial for optimisation problems and machine learning algorithms.
For instance, gradient descent, a popular optimisation algorithm used in machine learning, relies heavily on calculus.
By understanding the principles of calculus, data scientists can fine-tune these algorithms and improve their performance.
Math in data science: linear algebra
Linear algebra is a branch of mathematics that deals with vectors, vector spaces, and linear transformations.
It is essential for understanding and implementing machine learning algorithms, as many of these algorithms operate on data in vector spaces.
For example, linear regression, a common machine learning algorithm, is based on the principles of linear algebra.
By understanding these principles, data scientists can better understand how these algorithms work and how to use them effectively.
Math in data science: probability theory
Probability theory is a branch of mathematics that deals with uncertainty.
It is used in data science to model and understand random phenomena, make predictions, and make decisions under uncertainty.
For instance, Bayesian inference, a method of statistical inference, is based on probability theory.
By understanding the principles of probability, data scientists can make more accurate predictions and better decisions based on data.
Conclusion
In conclusion, how much math is in data science is complex.
The amount of math in data science can vary depending on the specific role and the nature of the work.
However, at its core, data science heavily relies on several key areas of mathematics: statistics, calculus, linear algebra, and probability theory.
Understanding these mathematical disciplines and their use in data science is crucial for anyone considering a career in this field.
By delving into these areas, one can gain a deeper understanding of the mathematical foundations of data science and be better equipped to tackle its challenges.
Are you passionate about data science?
The Institute of Data’s Data Science & AI program offers practical training in data analytics, machine learning, and AI.