Putting Data to the Test: A Guide to Statistical Testing in Practice
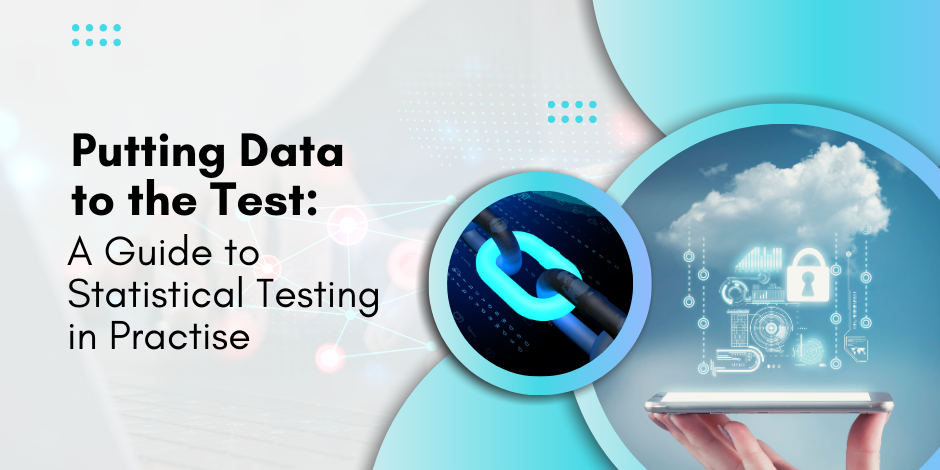
Stay Informed With Our Weekly Newsletter
Receive crucial updates on the ever-evolving landscape of technology and innovation.
Statistical testing is a crucial data analysis component that allows researchers to make informed decisions based on evidence.
We can use statistical tests to determine the significance of relationships, differences, or trends within data sets.
Understanding the basics of statistical testing
What is statistical testing?
Statistical testing involves applying mathematical models and techniques to assess the likelihood of observed differences in data being due to chance.
It helps us determine whether the relationships or patterns we observe are statistically significant, meaning they are unlikely to have occurred by chance alone.
Statistical testing is fundamental in various fields, including science, economics, and social sciences.
Researchers use statistical tests to analyse data and draw meaningful conclusions, ensuring their findings are robust and reliable.
Researchers can uncover hidden patterns, relationships, and trends within datasets by applying statistical methods, providing valuable insights into complex phenomena.
The importance of statistical testing in data analysis
Statistical testing is crucial because it allows us to draw conclusions from data and make educated decisions.
Without it, we would be left with incomplete or unreliable information.
By conducting appropriate statistical tests, we can determine the validity of our findings and ensure that our conclusions are supported by evidence.
Moreover, statistical testing helps researchers assess the impact of interventions or treatments in experimental studies.
Researchers can determine the effectiveness of a particular intervention by comparing outcomes between control and experimental groups using statistical tests.
This information is vital for evidence-based medicine, psychology, and public policy decisions.
Different types of statistical tests
Parametric tests and their applications
Parametric tests assume specific distributions and make certain assumptions about the data, such as normality and homogeneity of variances.
They are commonly used when the data meets these assumptions.
Examples of parametric tests include t-tests, analysis of variance (ANOVA), and regression analysis.
When conducting a t-test, researchers compare the means of two independent groups to determine if there is a statistically significant difference between them.
Conversely, ANOVA compares the means of three or more groups to see if they have a significant difference.
Regression analysis helps better understand the relationship between variables, allowing researchers to make predictions based on the data.
Non-parametric tests and when to use them
Non-parametric tests are used when the assumptions of parametric tests are violated.
These tests do not rely on distributional assumptions and are, therefore, more robust when the data do not meet the requirements of parametric tests.
Non-parametric tests include the Mann-Whitney U, Kruskal-Wallis, and Wilcoxon signed-rank tests.
The Mann-Whitney U test, also known as the Wilcoxon rank-sum test, compares two independent samples to assess if they are from the same population or if one tends to have higher values than the other.
The Kruskal-Wallis test extends the Mann-Whitney U test and compares more than two independent samples.
On the other hand, the Wilcoxon signed-rank test is used to compare two related samples and determine whether there is a significant difference between them.
Steps in conducting a statistical test
Formulating a hypothesis
Before conducting a statistical test, it is important to formulate a hypothesis.
A hypothesis is a statement that predicts the relationship or difference between variables. It helps guide our analysis and provides a framework for interpreting the results.
Formulating a hypothesis is a vital step in the scientific method. It allows researchers to make predictions and test them empirically.
A well-defined hypothesis provides clarity and direction to the study, ensuring that the research objectives are clearly outlined and the analysis is focused.
Choosing the right statistical test
Selecting the best statistical test is essential for obtaining accurate and meaningful results.
The choice of test depends on various factors, such as the nature of the data, the research question, and the assumptions that can be made.
Consulting statistical textbooks or software guides or seeking expert advice can help make the right choice.
Choosing the right statistical test requires understanding each test’s assumptions and requirements.
Researchers must consider the level of measurement of their variables, the distribution of the data, and the specific hypotheses being tested to ensure the chosen test is appropriate for the research question.
Interpreting the results of a statistical test
Interpreting these results involves assessing the findings’ statistical significance, effect size, and practical implications.
Understanding the meaning of p-values, confidence intervals, and other statistical measures is essential to draw valid conclusions from the analysis.
Interpreting the results of a statistical test requires a comprehensive analysis of the data in the context of the research question.
Researchers need to consider the results’ statistical significance, the effect’s impact, and its practical implications.
This holistic approach to interpretation ensures that the findings are statistically sound, relevant, and applicable in real-world scenarios.
Common pitfalls in statistical testing
Misinterpretation of p-values
Misinterpreting p-values is a common mistake in statistical testing.
A p-value represents the probability of obtaining the observed results (or more extreme), assuming the null hypothesis is true.
It does not measure the magnitude of an effect or the importance of a finding.
Remembering that p-values are not the be-all and end-all of statistical analysis is crucial.
They should be used with other measures of evidence and effect size to draw robust conclusions from data.
Researchers must exercise caution in interpreting p-values and avoid drawing definitive conclusions based solely on them.
Over-reliance on statistical significance
Statistical significance does not always imply practical significance.
Researchers need to consider the effect size, confounding factors, and the context of the research question.
Relying solely on statistical significance can lead to misleading conclusions and overlook important relationships or patterns.
Moreover, statistical significance is influenced by sample size, and a large sample size can make even trivial effects statistically significant.
Therefore, it is essential to interpret statistical significance in the context of the research question and the findings’ practical implications.
Researchers should aim for a balance between statistical rigour and practical relevance in their analyses.
Advanced statistical testing techniques
Multivariate analysis: An overview
Multivariate analysis involves studying the relationships between three or more variables simultaneously.
It enables researchers to investigate complex interactions and make predictions based on multiple factors.
Common multivariate techniques include factor analysis, cluster analysis, and structural equation modelling.
Time series analysis and its uses
Time series analysis focuses on analysing data that changes over time. It helps researchers understand trends and patterns and predict future values.
Time series techniques include autoregressive integrated moving average models, exponential smoothing, and forecasting methods.
Conclusion
Statistical testing is an essential tool in data analysis. It allows us to draw meaningful conclusions and make informed decisions based on evidence.
By understanding the basics, different types of tests, and steps involved and avoiding common pitfalls, researchers can ensure the accuracy and validity of their findings.
Furthermore, advanced techniques such as multivariate and time series analysis offer more comprehensive approaches to analysing complex data sets.
Mastering statistical testing techniques is crucial for researchers in various fields. It provides them with the necessary skills to analyse and interpret data confidently.
Want to advance your data science career? The Institute of Data’s Data Science & AI program offers an in-depth, comprehensive curriculum for beginners and seasoned professionals.
Nurture your passion for data science with a supportive environment, resources and flexible learning to suit your timetable. Ready to learn more about our programs? Contact our local team for a free career consultation.