What is Fog Data Science?
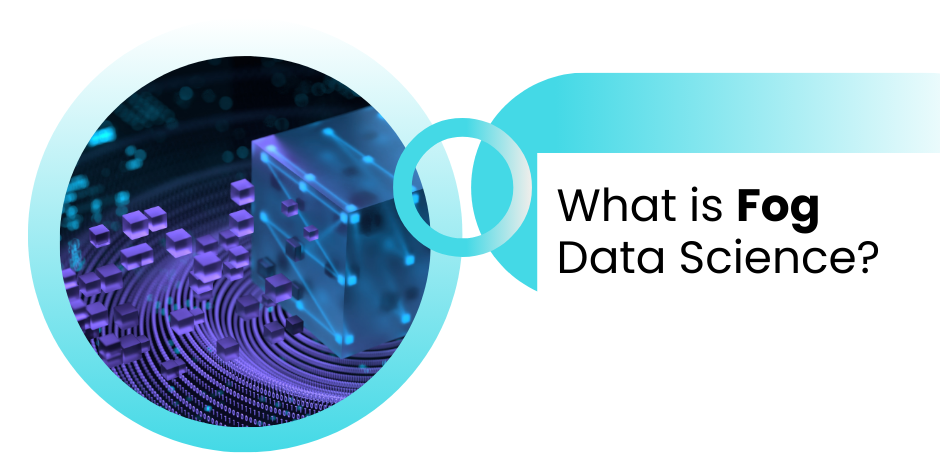
Stay Informed With Our Weekly Newsletter
Receive crucial updates on the ever-evolving landscape of technology and innovation.
In the ever-evolving world of data science, new concepts and methodologies are constantly emerging.
Amidst the rapidly expanding data landscape, statistics for 2024 estimate a staggering 149 zettabytes of data to be copied, captured, and curated.
This exponential growth is monumental compared to the mere two zettabytes generated back in 2010, indicating a relentless surge in global data.
One concept at the forefront of this data revolution is fog data science.
But what exactly is it?
This article aims to provide a comprehensive understanding of fog data science against the backdrop of this data explosion.
Defining fog data science
Fog data science, also known as fog computing, is a decentralised computing infrastructure in which data, computing, storage and applications are distributed in the most logical, efficient place between the data source and the cloud.
It’s a system-level horizontal architecture that distributes resources and services of computing, storage, control, and networking anywhere from the centralised cloud (remote data processing) to the edge devices, commonly referred to as “Things” (such as Internet of Things devices or sensors at the source of data).
The term ‘fog’ is a metaphor for a layer of cloud close to the ground, implying that decision-making (data processing) is done at a local level rather than in a remote location such as the cloud.
This is the fundamental principle of fog.
The role of fog data science
Fog data science plays a crucial role in managing the massive amounts of data generated by Internet of Things (IoT) devices.
These devices produce a vast amount of data that needs to be processed quickly for real-time or near-real-time decision-making.
Sending all this data to the cloud for processing can be time-consuming and resource-intensive.
This is where it comes into play.
By processing data closer to its source, it reduces the amount of data that needs to be sent to the cloud, thereby reducing transmission costs and latency.
This is particularly beneficial for applications that require real-time processing and analytics.
Applications and uses
There are numerous applications of fog data science across various industries.
In healthcare, for instance, fog computing can be used to process data from wearable devices in real-time, enabling immediate feedback for patients and healthcare providers.
In the transportation industry, it can be used for real-time traffic management and predictive maintenance of vehicles.
Another significant application of fog data science is in smart cities.
Here, fog computing can be used to process data from various sensors and devices in real-time, enabling efficient management of resources and services.
From traffic management to energy use, it can help make cities smarter and more efficient.
Benefits of fog data science
Fog data science offers several benefits over traditional cloud computing.
Firstly, by processing data closer to its source, fog computing reduces latency, which is crucial for applications that require real-time processing.
This can significantly improve the performance of these applications and enhance user experience.
Secondly, fog data science can reduce transmission costs by minimising the amount of data that needs to be sent to the cloud.
This can result in significant cost savings, particularly for organisations that generate large amounts of data.
Finally, fog data science can enhance security and privacy by keeping sensitive data closer to its source.
This can be particularly beneficial for applications that handle sensitive data, such as healthcare or financial services.
Challenges of implementing fog data science
Despite its numerous benefits, implementing fog data science is not without its challenges.
One of the key challenges is the need for significant investment in infrastructure.
Implementing fog computing requires a robust network infrastructure to support data distribution, computing, storage and applications.
Another challenge is the complexity of managing a distributed computing environment.
This requires sophisticated management and orchestration tools, as well as skilled information technology (IT)network personnel.
Finally, security is a major concern in fog data science.
While keeping data closer to its source can enhance security, it also presents new security challenges.
These include securing the data in transit and ensuring the integrity of the data at the edge.
Conclusion
Fog data science is a promising concept that offers significant benefits, particularly for applications that require real-time processing and analytics.
However, implementing fog computing requires careful consideration of the associated challenges and costs.
With the right approach and investment, fog data science can provide a powerful tool for managing the massive amounts of data generated in our increasingly connected world.
Learn more about data science by exploring the Institute of Data’s comprehensive Data Science & AI programme.
Alternatively, we invite you to schedule a complimentary career consultation with a member of our team to discuss the programme in more detail.