Data Theory vs Data Science: What’s the Difference?
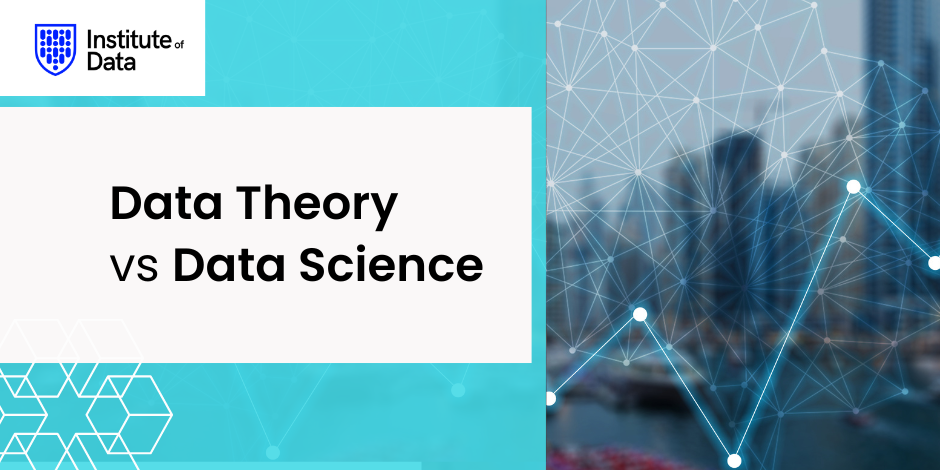
Stay Informed With Our Weekly Newsletter
Receive crucial updates on the ever-evolving landscape of technology and innovation.
In today’s landscape of data-driven decision-making, it’s common to hear the terms data theory vs data science.
While often used interchangeably, these two fields are distinct, each with its unique set of principles, methodologies, and applications.
Recent statistics underscore the edge that data-driven companies have over their peers.
According to a detailed study by BPM and Wintergreen Research, such companies are notably more likely to adopt business intelligence systems for data access and reporting, being 7.5 times more inclined to do so.
They are also 5.2 times more likely to use marketing automation systems that rely on digital data.
This shift towards data-focused strategies means these companies base their decisions on analytics rather than intuition, leading to markedly improved performance across various metrics.
This trend highlights the growing importance of data theory vs data science.
While data theory lays the groundwork for understanding data at a fundamental level, data science brings this knowledge to life, applying it to drive tangible business outcomes.
Together, they form the backbone of successful data-driven decision-making in the modern business environment, and understanding their differences is essential for anyone looking to thrive in the complex world of data-driven strategies.
Defining the concepts: data theory vs data science
What is data theory?
Data Theory is a branch of mathematics that focuses on the formalisation and analysis of data structures, algorithms, and complexity.
It encompasses various concepts such as data modelling, data management, and data analysis.
Data theory aims to develop rigorous frameworks for understanding the fundamental principles underlying the manipulation and interpretation of data.
Data theory delves into the intricacies of data representation and processing, exploring the mathematical foundations that underpin the storage and retrieval of information.
By studying data structures and algorithms, researchers in this field seek to enhance the efficiency and effectiveness of data manipulation techniques.
Through the lens of data theory, analysts can gain a deeper understanding of how data is organised and utilised in various computational processes.
What is data science?
Data science, on the other hand, is an interdisciplinary field that combines scientific methods, processes, algorithms, and systems to extract knowledge and insights from structured and unstructured data.
It involves the study of data analysis, machine learning, statistical modelling, and data visualisation techniques.
Data science is primarily concerned with extracting actionable intelligence from vast amounts of data to drive informed decision-making.
Within the realm of data theory vs data science, professionals leverage advanced analytical tools and techniques to uncover patterns and trends hidden within complex datasets.
By harnessing the power of statistical algorithms and machine learning models, Data Scientists can derive valuable insights that inform strategic business decisions and predictive analytics.
The interdisciplinary nature of data theory vs data science fosters collaboration across domains, enabling experts to combine expertise in computer science, mathematics, and domain-specific knowledge to extract meaningful information from data.
The historical context of data theory
Data theory has its roots in classical mathematics and logic, with pioneers such as Alan Turing and Claude Shannon making significant contributions.
Throughout the 20th century, advancements in computer science and information theory propelled data theory forward, paving the way for the modern understanding of data structures and algorithms.
The rise of data science
Data science emerged as a distinct field in the late 20th century, driven by the increasing availability of data and the need to extract meaningful insights.
The advent of powerful computers and the proliferation of digital technologies fuelled the growth of data science, enabling researchers and practitioners to tackle complex problems and uncover hidden patterns in large datasets.
The impact of data theory on modern technology
With the foundation laid by data theory, modern technology has been revolutionised.
From artificial intelligence and machine learning to data mining and predictive analytics, data theory underpins the algorithms and models that power these cutting-edge technologies.
By understanding the principles of data theory vs data science, developers and data scientists can create innovative solutions that drive progress in various industries.
The interdisciplinary nature of data science
One of the key characteristics of data science is its interdisciplinary nature.
Drawing insights from fields such as statistics, computer science, and domain-specific knowledge, data theory vs data science combines various disciplines to extract knowledge and insights from data.
This interdisciplinary approach not only enriches the field of data science but also enables a holistic understanding of complex datasets and phenomena.
Key differences between data theory and data science
One key distinction between data theory vs data science lies in their approach and methodologies.
Data theory focuses on developing formal frameworks and mathematical models to represent and analyse data structures, while data science employs a more practical, empirical approach, utilising statistical techniques, machine learning algorithms, and data visualisation tools to gain insights from data.
Data theory also primarily serves as a foundation for data science, providing the theoretical underpinnings and principles upon which the practice of data science is built.
While data theory is concerned with the abstract and theoretical aspects of data, data science is concerned with applying these principles to solve real-world problems and drive decision-making in various domains, such as business, healthcare, finance, and social sciences.
How data theory informs data science
Data theory plays a vital role in shaping the practice of data science.
By providing a rigorous framework for understanding and manipulating data, Data theory enables Data Scientists to develop robust algorithms, design efficient data structures, and make sound statistical inferences.
Data theory also helps Data Scientists understand the limitations and assumptions underlying various data analysis techniques, ensuring the validity and reliability of their results.
The interplay between theory and practice
There is a symbiotic relationship between data theory vs data science.
While data theory provides the theoretical foundations, data science serves as a testing ground for validating and refining these theories.
Through practical experimentation, Data Scientists gain insights into the practical challenges of working with real-world data and use this knowledge to inform and enhance data theory.
Skills required for data theory and data science
Essential skills for a Data Theorist
For a Data Theorist, a strong mathematical foundation is essential. Proficiency in areas such as linear algebra, probability theory, and algorithms is crucial.
Additionally, a deep understanding of formal logic, computational complexity theory, and data modelling techniques is necessary to excel in this field.
Essential skills for a Data Scientist
A Data Scientist, on the other hand, needs a diverse skill set.
Proficiency in statistical analysis, machine learning, and programming languages such as Python and R is indispensable.
Data visualisation, data preprocessing, and domain knowledge are also crucial for extracting actionable insights and communicating findings effectively.
Conclusion
Although data theory vs data science are closely related, they represent distinct disciplines with their own unique contributions to the field of data and analytics.
By understanding the differences between data theory vs data science, individuals can make more informed choices when pursuing a career or engaging with data-driven projects.
To gain both the education and the experience you need in the field of Data Science, consider exploring the Institute of Data’s comprehensive Data Science & AI programme.
Alternatively, we invite you to schedule a complimentary career consultation with a member of our team to discuss the programme in more detail.