Maths in Data Science: How Much is Required?
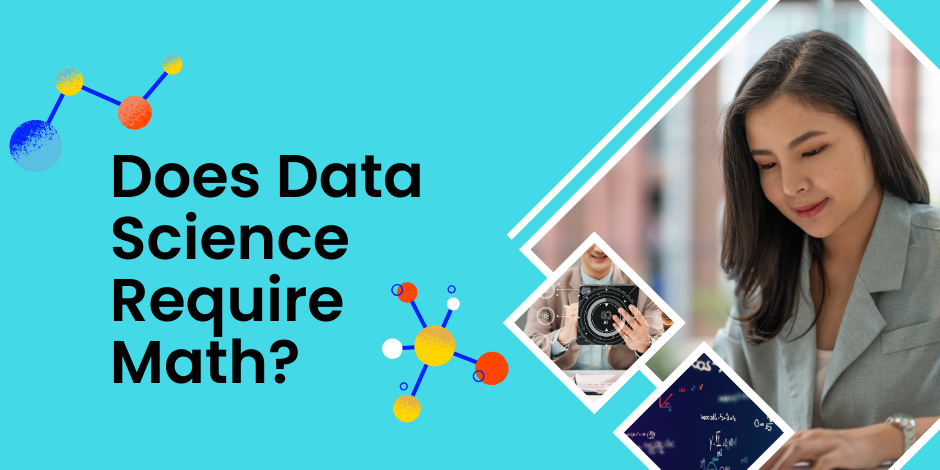
Stay Informed With Our Weekly Newsletter
Receive crucial updates on the ever-evolving landscape of technology and innovation.
As the world becomes increasingly data-driven, data science has emerged as a critical area of expertise.
This multidisciplinary field combines statistics, computer science, and business intelligence to extract meaningful insights from raw data.
A common question among those considering a career in data science is – “When it comes to maths in data science, how much is required?”
The short answer is that data science does require a solid understanding of mathematics.
However, the extent and depth of mathematical knowledge required can vary depending on the specific role and the nature of the work involved.
This article will delve into maths in data science.
We’ll explore why maths in data science is important, what areas of maths are most relevant, and how to build the necessary mathematical skills for a career in data science.
Why does data science require mathematics?
At its core, data science is about making sense of data.
This involves identifying patterns, making predictions, and drawing conclusions from large and often complex data sets.
Mathematics provides the tools and scaffolding necessary to perform these tasks effectively and accurately.
Mathematics is the language of precision and logic.
It allows data scientists to formulate hypotheses, construct models, and test predictions rigorously and systematically.
With a solid mathematical foundation, performing these tasks with any degree of confidence or accuracy would be easier.
Furthermore, many algorithms and techniques used in data science are based on mathematical concepts.
For example, machine learning algorithms, a key component of many data science projects, are built on statistics, calculus, and linear algebra principles.
Understanding these mathematical foundations can help data scientists develop more effective algorithms and better use existing ones.
What areas of mathematics are relevant to data science?
While maths in data science is required, it’s important to note that not all areas of maths are equally relevant.
The three main areas of mathematics most applicable to data science are statistics, calculus, and linear algebra.
Maths in data science: Statistics
Statistics is the most directly relevant area of mathematics for data science. It provides:
- The tools for understanding data.
- Making predictions.
- Making decisions based on data.
This includes probability, regression, hypothesis testing, and Bayesian inference.
Moreover, statistics is at the heart of many data science techniques, including machine learning.
Understanding statistical principles can help data scientists choose the right models, interpret their results, and avoid common pitfalls.
Maths in data science: Calculus
Calculus is another important area of mathematics for data science.
It is used in optimisation problems, a common task in machine learning and data analysis.
For example, gradient descent, a popular optimisation algorithm, is based on concepts from calculus.
Calculus also provides the foundation for understanding how changes in one variable can affect others, which is a key aspect of many data science problems.
Maths in data science: Linear Algebra
Linear algebra is the study of vectors and matrices.
It is used extensively in machine learning and data analysis, particularly in areas such as image processing, natural language processing, and deep learning.
Understanding linear algebra can help data scientists work more effectively with large, high-dimensional data sets and develop more efficient algorithms.
Building mathematical skills for data science
Given the importance of maths in data science, aspiring data scientists need to build strong mathematical skills.
This can be achieved through self-study, formal education, or both.
Many universities offer statistics, calculus, and linear algebra courses, which can provide a solid foundation for a career in data science.
A popular alternative path to enter the data science industry is through accredited programs such as those offered by the Institute of Data.
Online courses and tutorials can also be a valuable resource, particularly for those who prefer to learn at their own pace or who want to supplement their formal education.
Practical experience is also essential. Applying mathematical concepts to real-world data science problems can help reinforce learning and develop practical skills.
This can be achieved through projects, internships, or work experience in a data science role.
Conclusion
While maths in data science is essential, the depth and breadth of mathematical knowledge required can vary.
A solid understanding of statistics, calculus, and linear algebra can provide a strong foundation for a career in data science.
By building these skills and applying them to real-world problems, aspiring data scientists can prepare themselves for a successful career in this exciting and rapidly evolving field.
Are you new to data science or transitioning into this dynamic career?
The Institute of Data’s Data Science & AI program opens a world of opportunity with cutting-edge theoretical knowledge and practical application.
Our industry-expert trainers will get you job-ready and equipped to tackle real-world challenges in this ever-evolving field of tech.
Want to learn more about our programs? Contact our local team for a free career consultation.