How Long Does It Take to Learn Data Science?
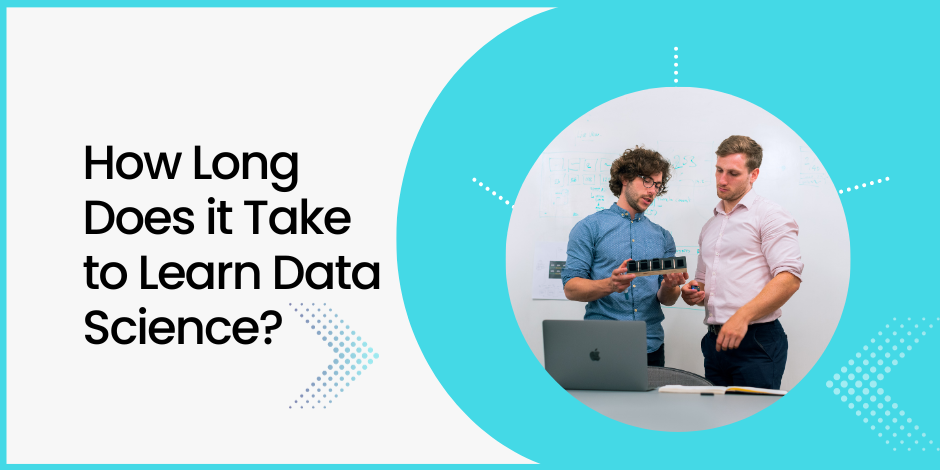
Stay Informed With Our Weekly Newsletter
Receive crucial updates on the ever-evolving landscape of technology and innovation.
Data science has become increasingly popular in recent years, with its applications spanning various industries.
Aspiring professionals are often curious about the time it takes to master the skills required for a career in data science.
While there is no definitive answer to this question, understanding the basics, the journey to becoming a data scientist, time investment, and the different specialisations can provide some insights.
The basics of data science
Data science is a multidisciplinary field that involves mathematics, programming, and domain expertise to derive actionable insights from data.
To embark on a data science journey, it is essential to have a strong foundation in mathematics and a solid understanding of programming languages.
The role of mathematics
Mathematics plays a vital role in data science.
Understanding statistics will enable you to make informed decisions based on data-driven insights. Statistics involves collecting, analysing, interpreting, and presenting data.
In addition to statistics, linear algebra is another mathematical concept that is fundamental to data science. It deals with vectors, matrices, and linear transformations.
Linear algebra is used in various data science tasks, such as dimensionality reduction, clustering, and machine learning algorithms.
Having a solid understanding of linear algebra will allow you to manipulate and analyse data efficiently.
Calculus, on the other hand, focuses on the study of change and motion.
In data science, calculus is used for optimisation problems, such as finding the maximum or minimum of a function.
It is also used in machine learning algorithms, where gradient descent is a common optimisation technique.
Understanding calculus will help you optimise models and algorithms to achieve better performance.
The most used programming languages
Familiarising yourself with programming languages is another crucial aspect of learning data science.
Python, with its clean syntax and extensive libraries, has become the go-to language for data scientists.
It provides a wide range of tools for data manipulation, analysis, and visualisation.
Python’s simplicity makes it accessible to beginners, while its versatility allows experienced programmers to build complex data science pipelines.
R, on the other hand, is a language specifically designed for statistical analysis and data visualisation.
It has a vast collection of packages that cater to various data science needs.
R’s strong statistical capabilities make it a preferred choice for researchers and statisticians.
With R, you can efficiently perform advanced statistical analysis and create visually appealing plots and charts.
The journey to becoming a data scientist
Your primary responsibility is to extract, clean, and analyse data to generate valuable insights that drive organisation decision-making.
Proficiency in database management, data visualisation, and machine learning is also essential to succeed in this field.
Database management skills are crucial for efficiently handling and manipulating large datasets.
You will learn how to design and optimise databases, ensuring data is organised, accessible, and secure.
Understanding the intricacies of data storage and retrieval mechanisms equips you with the ability to navigate through complex data landscapes.
Data visualisation is another vital skill that you will master along your journey.
As a data scientist, you will often be tasked with presenting complex information in a visually appealing and easily understandable manner.
Using charts, graphs, and interactive dashboards, you will bring data to life, enabling stakeholders to grasp key insights effortlessly.
Machine learning is the backbone of modern data science. You will dive deep into the world of algorithms, understanding how they work and when to apply them.
Whether it’s classification, regression, or clustering, you will become well-versed in the art of modelling and predicting outcomes from data.
However, technical skills alone are insufficient to thrive as a data scientist.
The ability to communicate effectively is equally essential.
As you progress on your journey, you will develop strong storytelling skills, enabling you to convey complex concepts and findings to technical and non-technical audiences.
You will become a master of translating data into actionable insights that drive meaningful change.
Moreover, becoming a data scientist is not limited to acquiring technical skills.
It is a continuous process of staying updated with the latest advancements in the field.
As technology evolves quickly, you must remain curious and embrace lifelong learning.
Attending conferences, participating in online communities, and engaging in collaborative projects will help you stay at the forefront of this ever-evolving field.
Time investment in learning data science
When learning data science, two options are commonly considered: self-study and formal education.
Self-study allows individuals to learn at their own pace and explore specific areas of interest.
On the other hand, formal education, such as a degree or a boot camp, provides a more structured learning path and access to mentors.
Regardless of the chosen approach, time management and learning strategies are vital in mastering data science skills.
Consistency is critical, as dedicating regular time to practice and solve real-world problems is essential to reinforcing learning.
Breaking complex concepts into manageable sections and gradually building upon them can help solidify understanding.
Delving deeper into data science specialisations
Two prominent specialisations are machine learning, artificial intelligence (AI) and big data.
Machine learning involves training algorithms to learn from data and make predictions or decisions. AI, on the other hand, focuses on creating intelligent systems that can mimic human intelligence.
Big data refers to the vast and complex datasets.
With the exponential growth of data, organisations are seeking professionals with expertise in big data technologies such as Hadoop and Spark.
Understanding the infrastructure and tools required to handle big data is a valuable skill in the data science landscape.
Career prospects in data science
Data science has emerged as one of the most promising career paths in recent years.
The job market for data scientists is highly lucrative, with organisations across various sectors, including finance, healthcare, and technology, actively seeking skilled professionals.
As businesses increasingly rely on data-driven decision-making, data scientists are in high demand.
Conclusion
The time it takes to learn data science depends on various factors such as prior knowledge, learning approach, and dedication.
A solid foundation in mathematics and programming languages and practical experience can expedite the learning process.
By continually expanding knowledge and staying up-to-date with advancements, one can embark on a successful career in data science.
Want to dive into data science? The Institute of Data offers industry-relevant training to stay competitive in this dynamic area.
Choose the Institute of Data as your learning partner to equip yourself with the skills and expertise needed to succeed.
Keen to learn more? Contact our local team for a free career consultation.