How Siddhartha Upskilled into Data Science & AI to Boost his Career Prospects
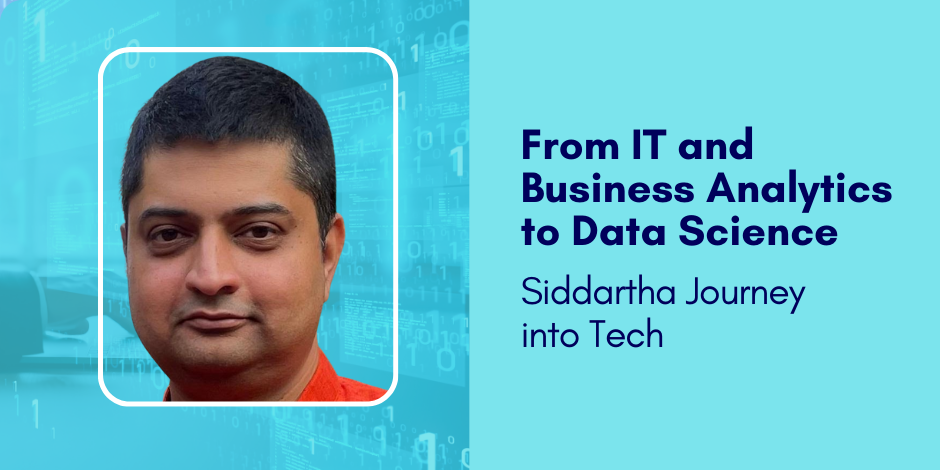
Stay Informed With Our Weekly Newsletter
Receive crucial updates on the ever-evolving landscape of technology and innovation.
With over 17 years of domain knowledge and industry experience in information technology (IT) and business analytics, Siddhartha was determined to secure a Data Science Job in Melbourne, Australia.
He balanced a full-time job with part-time learning and was trained by the industry experts at the Institute of Data in just 24 weeks through the part-time Data Science & Artificial Intelligence (AI) Program.
This additional study allowed him to leverage his existing skillset and expand his career prospects.
This is his journey so far:
1. Siddartha, what motivated you to upskill in the Data Science and AI industry?
I have over 17 years of experience in the IT world.
Since the beginning of my career, I was always interested in learning new things.
As a result, I have engaged in multiple roles – such as a solution architect, business analyst and even presales consultant.
With Industry 4.0 (the ‘fourth’ phase of the industrial revolution), data and applications around data and insights have become essential for any decision-making in any industry.
It is now essential to be data-literate.
In the future, my dream is to become an AI product owner – and this is why I decided to study the Data Science & AI Program.
2. How did you find the program overall?
I work a full-time job.
So, balancing my work and part-time studies was the most challenging part.
It was quite a stretch, and I want to thank my wife for supporting me throughout this journey.
The most enjoyable part of the program was attending the classes, just like I had done last in my MBA days.
3. Having experience in the IT field already, how did you transfer and apply those experiences to apply for data science jobs?
For any new learning area, it is important to understand the fundamentals and the building blocks.
My experience has taught me to figure out those fundamentals and build a strong foundation on them – I believe that helped me in my learning journey.
Data science jobs are quite broad, and it is very easy to get lost in the overwhelming information overload.
So, having a step-by-step approach always helps one grasp the subject.
Once the foundation is strong, it becomes easier and one can learn more deeply within any area.
Another aspect that helped me was the understanding of programming languages & SQL.
These are essential in the data world.
Although I am not a developer, it is good to have that skill and Python as the language was easy to learn.
4. What were your career goals before upskilling to data and how has your outlook changed?
Well, I had a goal of moving to product management.
Being part of multiple transformation programs globally, I have the drive to become a full-fledged product manager.
After having studied the Data Science & AI program, that goal has shifted towards becoming a product manager for an AI product.
The core underlying concept remains the same; only the work area is now more channelised.
5. Why did you choose to join the Part-Time Program in particular?
As I work a full-time job, the part-time format was the most suitable for me.
The most appealing part is the face-to-face learning – I simply loved it.
6. Tell us about your capstone project. How did you come up with your topic?
Finding the right topic for the capstone project was the most challenging part.
You must have a problem statement and the underlying data in the data world.
Both are equally important to make the project successful.
My topic was on predicting how soon a customer request can be fulfilled.
We often see customers log service requests with service providers, and we get a blanket message that it will be done within X days.
As a consumer, this does not help, especially for services that need immediate attention.
The underlying data was service request data of NYC 311.
This is one of the largest service providers in the city of New York – serving ~10Mn people.
It is essential to identify the business objective before solving a data problem.
From the same data, multiple dimensions can be analysed and solved.
At the end of the day, if it does not yield business value, then that outcome is of no use.
Driving the data problem from the business problem and aligning the outcome to business KPIs is the best part of the project.
7. How did you find the process of completing your final Capstone project during the program?
Completing my capstone project taught me so many important and valuable lessons.
It was important for me to start early – define the problem statement and the underlying data as time does fly quickly.
I noticed the problem statement and underlying data could not actually match, so it was important for me to be flexible in tweaking the problem statement without compromising the business value.
In addition, I noticed many individuals get bogged down by the level of accuracy in their projects.
I found it important to not be disheartened, the learning is the important aspect, not the accuracy.
Finally, I discovered the necessity to keep experimenting till the end and make time for documentation and presentation preparation.
[su_quote]The overall true beauty of data science is the necessity to experiment to the end, which I continually enjoy.[/su_quote]8. What advice would you give someone developing their Capstone project during the program?
Some of the learnings are as follows –
- Start early on defining the problem statement & the underlying data. Time flies very quickly.
- It might so happen that the problem statement & underlying data may not match. Tweak the problem statement without compromising business value.
- Data is often not clean enough. Data cleaning is a prerequisite for any machine learning (ML) problem. So, allot time for that activity.
- People sometimes get bogged down on the accuracy levels. Well, you need to understand it is a capstone project and not something you will run on the production. Don’t get disheartened. You will get ample opportunity in real life to match high accuracy levels. Accuracy is not that important, the learning is more important.
- Keep experimenting till the end. You never know what will happen if you don’t experiment.
- Finally, keep time for documentation and presentation preparation. It is equally important for the completion of the project.
9. What did you enjoy the most about the Capstone project?
Performing while learning is a key part of the journey.
The program was divided into multiple modules and each module had its own significance.
I believe almost all the modules have some relation to the capstone project.
Both the program and the capstone project were running in parallel, which is a unique method of teaching.
When the program ended, the capstone also came to an end.
This was a unique method and practical learning for me.
10. What tips would you give someone preparing to present their Capstone project at the end of the program?
For the presentation section, it is always important to engage the audience.
One needs to narrate a story.
No one is interested in what you coded or what language you have used.
While technical information is important, you must link the business problem to the project’s outcome.
Ultimately, people appreciate the value delivered.
Some presentation tips are:
- Have a clearly defined business problem to start with
- Highlight the solution approach – the various steps followed with some rational
- Highlight the key features of the data set used
- Highlight any challenges encountered & any mitigation strategy
- Finally, what is the result? Don’t stop on the accuracy of an ML model; highlight how the model adds value to the business.
11. What do you enjoy the most about data science, data analytics, machine learning & AI?
[su_quote]Data is the new oil. In the next decade, data science jobs will focus on the true value of data. With new technologies, the data space has become even more exciting. The hidden insights within data are of immense value to any business, and it’s an interesting journey to be part of the data world. [/su_quote]12. Do you want to add anything else or have any final thoughts?
I want to comment that a single program is not the end of learning.
One needs to be a lifelong learner in this world.
So consider this program as your stepping stone in the world of data.
Edit: As of 2024, Siddartha works as a Senior Data & AI Manager for Deloitte.
Connect with Siddhartha on LinkedIn here.
If you want to learn more about data science to boost your career prospects, consider reading the Institute of Data’s Data Science & AI Course Outline.
If you would like to discuss the program in more detail, book a free career consultation with a member of our team to see if the program is right for your situation.